rumale 0.22.0 → 0.22.5
Sign up to get free protection for your applications and to get access to all the features.
- checksums.yaml +4 -4
- data/.coveralls.yml +1 -0
- data/.github/workflows/build.yml +6 -3
- data/.github/workflows/coverage.yml +28 -0
- data/.gitignore +1 -0
- data/.rubocop.yml +1 -0
- data/CHANGELOG.md +35 -0
- data/Gemfile +6 -4
- data/LICENSE.txt +1 -1
- data/README.md +56 -19
- data/ext/rumale/tree.c +24 -12
- data/lib/rumale.rb +8 -0
- data/lib/rumale/base/base_estimator.rb +5 -3
- data/lib/rumale/dataset.rb +7 -3
- data/lib/rumale/decomposition/pca.rb +1 -1
- data/lib/rumale/ensemble/stacking_classifier.rb +215 -0
- data/lib/rumale/ensemble/stacking_regressor.rb +163 -0
- data/lib/rumale/ensemble/voting_classifier.rb +126 -0
- data/lib/rumale/ensemble/voting_regressor.rb +82 -0
- data/lib/rumale/feature_extraction/feature_hasher.rb +1 -1
- data/lib/rumale/feature_extraction/hash_vectorizer.rb +1 -1
- data/lib/rumale/kernel_approximation/nystroem.rb +29 -9
- data/lib/rumale/kernel_machine/kernel_ridge_classifier.rb +92 -0
- data/lib/rumale/kernel_machine/kernel_svc.rb +4 -3
- data/lib/rumale/linear_model/elastic_net.rb +1 -1
- data/lib/rumale/linear_model/lasso.rb +1 -1
- data/lib/rumale/linear_model/linear_regression.rb +63 -34
- data/lib/rumale/linear_model/logistic_regression.rb +1 -1
- data/lib/rumale/linear_model/nnls.rb +137 -0
- data/lib/rumale/linear_model/ridge.rb +70 -33
- data/lib/rumale/linear_model/svc.rb +4 -3
- data/lib/rumale/linear_model/svr.rb +4 -3
- data/lib/rumale/metric_learning/mlkr.rb +161 -0
- data/lib/rumale/metric_learning/neighbourhood_component_analysis.rb +7 -4
- data/lib/rumale/pairwise_metric.rb +1 -1
- data/lib/rumale/preprocessing/kernel_calculator.rb +92 -0
- data/lib/rumale/validation.rb +13 -1
- data/lib/rumale/version.rb +1 -1
- data/rumale.gemspec +1 -1
- metadata +14 -4
checksums.yaml
CHANGED
@@ -1,7 +1,7 @@
|
|
1
1
|
---
|
2
2
|
SHA256:
|
3
|
-
metadata.gz:
|
4
|
-
data.tar.gz:
|
3
|
+
metadata.gz: '058078489d3ff66d67432e1418ae786292c263e05e75b6703fb5a7e65e88bd46'
|
4
|
+
data.tar.gz: bd7ed9b223e0cd0074ffdd3e521b01c195f82909013c93f4736ab338d5920c96
|
5
5
|
SHA512:
|
6
|
-
metadata.gz:
|
7
|
-
data.tar.gz:
|
6
|
+
metadata.gz: 79ce4715a503b1b5a618526832adad5912daac72af3e8f1892ff2df14b7695e546419d6f85e5ea735abd2ea06da649a763f96c82b95eee75341934fa65fce93e
|
7
|
+
data.tar.gz: 5948583ec6c5ca10b320e09c447f9cc1e244dd3bfbf95800338dba2eb7e1b46a342d44020fa24c26a7161786feb63c82b0677654e4aa087c311337a606880a22
|
data/.coveralls.yml
ADDED
@@ -0,0 +1 @@
|
|
1
|
+
service_name: github-ci
|
data/.github/workflows/build.yml
CHANGED
@@ -1,13 +1,14 @@
|
|
1
1
|
name: build
|
2
2
|
|
3
|
-
on: [push]
|
3
|
+
on: [push, pull_request]
|
4
4
|
|
5
5
|
jobs:
|
6
6
|
build:
|
7
7
|
runs-on: ubuntu-latest
|
8
8
|
strategy:
|
9
|
+
fail-fast: false
|
9
10
|
matrix:
|
10
|
-
ruby: [ '2.5', '2.6', '2.7' ]
|
11
|
+
ruby: [ '2.5', '2.6', '2.7', '3.0' ]
|
11
12
|
steps:
|
12
13
|
- uses: actions/checkout@v2
|
13
14
|
- name: Install BLAS and LAPACK
|
@@ -17,7 +18,9 @@ jobs:
|
|
17
18
|
with:
|
18
19
|
ruby-version: ${{ matrix.ruby }}
|
19
20
|
- name: Build and test with Rake
|
21
|
+
env:
|
22
|
+
LD_LIBRARY_PATH: '/usr/lib/x86_64-linux-gnu/'
|
20
23
|
run: |
|
21
|
-
gem install bundler
|
24
|
+
gem install --no-document bundler
|
22
25
|
bundle install --jobs 4 --retry 3
|
23
26
|
bundle exec rake
|
@@ -0,0 +1,28 @@
|
|
1
|
+
name: coverage
|
2
|
+
|
3
|
+
on:
|
4
|
+
push:
|
5
|
+
branches: [ main ]
|
6
|
+
pull_request:
|
7
|
+
branches: [ main ]
|
8
|
+
|
9
|
+
jobs:
|
10
|
+
coverage:
|
11
|
+
runs-on: ubuntu-20.04
|
12
|
+
steps:
|
13
|
+
- uses: actions/checkout@v2
|
14
|
+
- name: Install BLAS and LAPACK
|
15
|
+
run: sudo apt-get install -y libopenblas-dev liblapacke-dev
|
16
|
+
- name: Set up Ruby 2.7
|
17
|
+
uses: actions/setup-ruby@v1
|
18
|
+
with:
|
19
|
+
ruby-version: '2.7'
|
20
|
+
- name: Build and test with Rake
|
21
|
+
run: |
|
22
|
+
gem install bundler
|
23
|
+
bundle install
|
24
|
+
bundle exec rake
|
25
|
+
- name: Coveralls GitHub Action
|
26
|
+
uses: coverallsapp/github-action@v1.1.2
|
27
|
+
with:
|
28
|
+
github-token: ${{ secrets.GITHUB_TOKEN }}
|
data/.gitignore
CHANGED
data/.rubocop.yml
CHANGED
data/CHANGELOG.md
CHANGED
@@ -1,3 +1,38 @@
|
|
1
|
+
# 0.22.5
|
2
|
+
- Add transformer class for calculating kernel matrix.
|
3
|
+
- [KernelCalculator](https://yoshoku.github.io/rumale/doc/Rumale/Preprocessing/KernelCalculator.html)
|
4
|
+
- Add classifier class based on Ridge regression.
|
5
|
+
- [KernelRidgeClassifier](https://yoshoku.github.io/rumale/doc/Rumale/KernelMachine/KernelRidgeClassifier.html)
|
6
|
+
- Add supported kernel functions to [Nystroem](https://yoshoku.github.io/rumale/doc/Rumale/KernelApproximation/Nystroem.html).
|
7
|
+
- Add parameter for specifying the number of features to [load_libsvm_file](https://yoshoku.github.io/rumale/doc/Rumale/Dataset.html#load_libsvm_file-class_method).
|
8
|
+
|
9
|
+
# 0.22.4
|
10
|
+
- Add classifier and regressor classes for voting ensemble method.
|
11
|
+
- [VotingClassifier](https://yoshoku.github.io/rumale/doc/Rumale/Ensemble/VotingClassifier.html)
|
12
|
+
- [VotingRegressor](https://yoshoku.github.io/rumale/doc/Rumale/Ensemble/VotingRegressor.html)
|
13
|
+
- Refactor some codes.
|
14
|
+
- Fix some typos on API documentation.
|
15
|
+
|
16
|
+
# 0.22.3
|
17
|
+
- Add regressor class for non-negative least square method.
|
18
|
+
- [NNLS](https://yoshoku.github.io/rumale/doc/Rumale/LinearModel/NNLS.html)
|
19
|
+
- Add lbfgs solver to [Ridge](https://yoshoku.github.io/rumale/doc/Rumale/LinearModel/Ridge.html) and [LinearRegression](https://yoshoku.github.io/rumale/doc/Rumale/LinearModel/LinearRegression.html).
|
20
|
+
- In version 0.23.0, these classes will be changed to attempt to optimize with 'svd' or 'lbfgs' solver if 'auto' is given to
|
21
|
+
the solver parameter. If you use 'sgd' solver, you need specify it explicitly.
|
22
|
+
- Add GC guard to native extension codes.
|
23
|
+
- Update API documentation.
|
24
|
+
|
25
|
+
# 0.22.2
|
26
|
+
- Add classifier and regressor classes for stacking method.
|
27
|
+
- [StackingClassifier](https://yoshoku.github.io/rumale/doc/Rumale/Ensemble/StackingClassifier.html)
|
28
|
+
- [StackingRegressor](https://yoshoku.github.io/rumale/doc/Rumale/Ensemble/StackingRegressor.html)
|
29
|
+
- Refactor some codes with Rubocop.
|
30
|
+
|
31
|
+
# 0.22.1
|
32
|
+
- Add transfomer class for [MLKR](https://yoshoku.github.io/rumale/doc/Rumale/MetricLearning/MLKR.html), that implements Metric Learning for Kernel Regression.
|
33
|
+
- Refactor NeighbourhoodComponentAnalysis.
|
34
|
+
- Update API documentation.
|
35
|
+
|
1
36
|
# 0.22.0
|
2
37
|
## Breaking change
|
3
38
|
- Add lbfgsb.rb gem to runtime dependencies. Rumale uses lbfgsb gem for optimization.
|
data/Gemfile
CHANGED
@@ -6,10 +6,12 @@ gemspec
|
|
6
6
|
gem 'mmh3', '>= 1.0'
|
7
7
|
gem 'numo-linalg', '>= 0.1.4'
|
8
8
|
gem 'parallel', '>= 1.17.0'
|
9
|
-
gem 'rake', '~>
|
9
|
+
gem 'rake', '~> 13.0'
|
10
10
|
gem 'rake-compiler', '~> 1.0'
|
11
11
|
gem 'rspec', '~> 3.0'
|
12
|
-
gem 'rubocop', '~> 0
|
12
|
+
gem 'rubocop', '~> 1.0'
|
13
13
|
gem 'rubocop-performance', '~> 1.8'
|
14
|
-
gem 'rubocop-
|
15
|
-
gem '
|
14
|
+
gem 'rubocop-rake', '~> 0.5'
|
15
|
+
gem 'rubocop-rspec', '~> 2.0'
|
16
|
+
gem 'simplecov', '~> 0.21'
|
17
|
+
gem 'simplecov-lcov', '~> 0.8'
|
data/LICENSE.txt
CHANGED
data/README.md
CHANGED
@@ -3,8 +3,9 @@
|
|
3
3
|
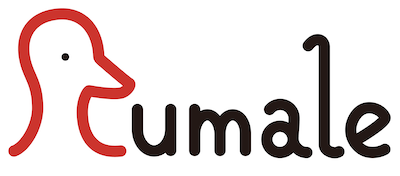
|
4
4
|
|
5
5
|
[](https://github.com/yoshoku/rumale/actions?query=workflow%3Abuild)
|
6
|
+
[](https://coveralls.io/github/yoshoku/rumale?branch=main)
|
6
7
|
[](https://badge.fury.io/rb/rumale)
|
7
|
-
[](https://github.com/yoshoku/rumale/blob/
|
8
|
+
[](https://github.com/yoshoku/rumale/blob/main/LICENSE.txt)
|
8
9
|
[](https://yoshoku.github.io/rumale/doc/)
|
9
10
|
|
10
11
|
Rumale (**Ru**by **ma**chine **le**arning) is a machine learning library in Ruby.
|
@@ -113,10 +114,10 @@ require 'rumale'
|
|
113
114
|
samples, labels = Rumale::Dataset.load_libsvm_file('pendigits')
|
114
115
|
|
115
116
|
# Define the estimator to be evaluated.
|
116
|
-
lr = Rumale::LinearModel::LogisticRegression.new
|
117
|
+
lr = Rumale::LinearModel::LogisticRegression.new
|
117
118
|
|
118
119
|
# Define the evaluation measure, splitting strategy, and cross validation.
|
119
|
-
ev = Rumale::EvaluationMeasure::
|
120
|
+
ev = Rumale::EvaluationMeasure::Accuracy.new
|
120
121
|
kf = Rumale::ModelSelection::StratifiedKFold.new(n_splits: 5, shuffle: true, random_seed: 1)
|
121
122
|
cv = Rumale::ModelSelection::CrossValidation.new(estimator: lr, splitter: kf, evaluator: ev)
|
122
123
|
|
@@ -124,15 +125,15 @@ cv = Rumale::ModelSelection::CrossValidation.new(estimator: lr, splitter: kf, ev
|
|
124
125
|
report = cv.perform(samples, labels)
|
125
126
|
|
126
127
|
# Output result.
|
127
|
-
|
128
|
-
puts
|
128
|
+
mean_accuracy = report[:test_score].sum / kf.n_splits
|
129
|
+
puts "5-CV mean accuracy: %.1f%%" % (100.0 * mean_accuracy)
|
129
130
|
```
|
130
131
|
|
131
132
|
Execution of the above scripts result in the following.
|
132
133
|
|
133
134
|
```bash
|
134
135
|
$ ruby cross_validation.rb
|
135
|
-
5-CV mean
|
136
|
+
5-CV mean accuracy: 95.4%
|
136
137
|
```
|
137
138
|
|
138
139
|
### Example 3. Pipeline
|
@@ -143,10 +144,10 @@ require 'rumale'
|
|
143
144
|
# Load dataset.
|
144
145
|
samples, labels = Rumale::Dataset.load_libsvm_file('pendigits')
|
145
146
|
|
146
|
-
# Construct pipeline with kernel approximation and
|
147
|
-
rbf = Rumale::KernelApproximation::RBF.new(gamma:
|
148
|
-
|
149
|
-
pipeline = Rumale::Pipeline::Pipeline.new(steps: { trns: rbf, clsf:
|
147
|
+
# Construct pipeline with kernel approximation and LogisticRegression.
|
148
|
+
rbf = Rumale::KernelApproximation::RBF.new(gamma: 1e-4, n_components: 800, random_seed: 1)
|
149
|
+
lr = Rumale::LinearModel::LogisticRegression.new(reg_param: 1e-3)
|
150
|
+
pipeline = Rumale::Pipeline::Pipeline.new(steps: { trns: rbf, clsf: lr })
|
150
151
|
|
151
152
|
# Define the splitting strategy and cross validation.
|
152
153
|
kf = Rumale::ModelSelection::StratifiedKFold.new(n_splits: 5, shuffle: true, random_seed: 1)
|
@@ -156,7 +157,7 @@ cv = Rumale::ModelSelection::CrossValidation.new(estimator: pipeline, splitter:
|
|
156
157
|
report = cv.perform(samples, labels)
|
157
158
|
|
158
159
|
# Output result.
|
159
|
-
mean_accuracy = report[:test_score].
|
160
|
+
mean_accuracy = report[:test_score].sum / kf.n_splits
|
160
161
|
puts("5-CV mean accuracy: %.1f %%" % (mean_accuracy * 100.0))
|
161
162
|
```
|
162
163
|
|
@@ -176,7 +177,7 @@ For example, using the [OpenBLAS](https://github.com/xianyi/OpenBLAS) speeds up
|
|
176
177
|
|
177
178
|
Install OpenBLAS library.
|
178
179
|
|
179
|
-
|
180
|
+
macOS:
|
180
181
|
|
181
182
|
```bash
|
182
183
|
$ brew install openblas
|
@@ -185,12 +186,13 @@ $ brew install openblas
|
|
185
186
|
Ubuntu:
|
186
187
|
|
187
188
|
```bash
|
188
|
-
$ sudo apt-get install
|
189
|
-
|
190
|
-
|
191
|
-
|
192
|
-
|
193
|
-
|
189
|
+
$ sudo apt-get install libopenblas-dev liblapacke-dev
|
190
|
+
```
|
191
|
+
|
192
|
+
Windows (MSYS2):
|
193
|
+
|
194
|
+
```bash
|
195
|
+
$ pacman -S mingw-w64-x86_64-ruby mingw-w64-x86_64-openblas mingw-w64-x86_64-lapack
|
194
196
|
```
|
195
197
|
|
196
198
|
Install Numo::Linalg gem.
|
@@ -206,6 +208,37 @@ require 'numo/linalg/autoloader'
|
|
206
208
|
require 'rumale'
|
207
209
|
```
|
208
210
|
|
211
|
+
### Numo::OpenBLAS
|
212
|
+
[Numo::OpenBLAS](https://github.com/yoshoku/numo-openblas) downloads and builds OpenBLAS during installation
|
213
|
+
and uses that as a background library for Numo::Linalg.
|
214
|
+
|
215
|
+
Install compilers for building OpenBLAS.
|
216
|
+
|
217
|
+
macOS:
|
218
|
+
|
219
|
+
```bash
|
220
|
+
$ brew install gcc gfortran make
|
221
|
+
```
|
222
|
+
|
223
|
+
Ubuntu:
|
224
|
+
|
225
|
+
```bash
|
226
|
+
$ sudo apt-get install gcc gfortran make
|
227
|
+
```
|
228
|
+
|
229
|
+
Install Numo::OpenBLAS gem.
|
230
|
+
|
231
|
+
```bash
|
232
|
+
$ gem install numo-openblas
|
233
|
+
```
|
234
|
+
|
235
|
+
Load Numo::OpenBLAS gem instead of Numo::Linalg.
|
236
|
+
|
237
|
+
```ruby
|
238
|
+
require 'numo/openblas'
|
239
|
+
require 'rumale'
|
240
|
+
```
|
241
|
+
|
209
242
|
### Parallel
|
210
243
|
Several estimators in Rumale support parallel processing.
|
211
244
|
Parallel processing in Rumale is realized by [Parallel](https://github.com/grosser/parallel) gem,
|
@@ -227,6 +260,10 @@ When -1 is given to n_jobs parameter, all processors are used.
|
|
227
260
|
estimator = Rumale::Ensemble::RandomForestClassifier.new(n_jobs: -1, random_seed: 1)
|
228
261
|
```
|
229
262
|
|
263
|
+
## Related Projects
|
264
|
+
- [Rumale::SVM](https://github.com/yoshoku/rumale-svm) provides support vector machine algorithms in LIBSVM and LIBLINEAR with Rumale interface.
|
265
|
+
- [Rumale::Torch](https://github.com/yoshoku/rumale-torch) provides the learning and inference by the neural network defined in torch.rb with Rumale interface.
|
266
|
+
|
230
267
|
## Novelties
|
231
268
|
|
232
269
|
* [Rumale SHOP](https://suzuri.jp/yoshoku)
|
@@ -244,4 +281,4 @@ The gem is available as open source under the terms of the [BSD 2-clause License
|
|
244
281
|
## Code of Conduct
|
245
282
|
|
246
283
|
Everyone interacting in the Rumale project’s codebases, issue trackers,
|
247
|
-
chat rooms and mailing lists is expected to follow the [code of conduct](https://github.com/yoshoku/Rumale/blob/
|
284
|
+
chat rooms and mailing lists is expected to follow the [code of conduct](https://github.com/yoshoku/Rumale/blob/main/CODE_OF_CONDUCT.md).
|
data/ext/rumale/tree.c
CHANGED
@@ -5,9 +5,8 @@ RUBY_EXTERN VALUE mRumale;
|
|
5
5
|
double*
|
6
6
|
alloc_dbl_array(const long n_dimensions)
|
7
7
|
{
|
8
|
-
long i;
|
9
8
|
double* arr = ALLOC_N(double, n_dimensions);
|
10
|
-
|
9
|
+
memset(arr, 0, n_dimensions * sizeof(double));
|
11
10
|
return arr;
|
12
11
|
}
|
13
12
|
|
@@ -257,10 +256,13 @@ find_split_params_cls(VALUE self, VALUE criterion, VALUE impurity, VALUE order,
|
|
257
256
|
split_opts_cls opts = { StringValuePtr(criterion), NUM2LONG(n_classes), NUM2DBL(impurity) };
|
258
257
|
VALUE params = na_ndloop3(&ndf, &opts, 3, order, features, labels);
|
259
258
|
VALUE results = rb_ary_new2(4);
|
260
|
-
|
261
|
-
rb_ary_store(results,
|
262
|
-
rb_ary_store(results,
|
263
|
-
rb_ary_store(results,
|
259
|
+
double* params_ptr = (double*)na_get_pointer_for_read(params);
|
260
|
+
rb_ary_store(results, 0, DBL2NUM(params_ptr[0]));
|
261
|
+
rb_ary_store(results, 1, DBL2NUM(params_ptr[1]));
|
262
|
+
rb_ary_store(results, 2, DBL2NUM(params_ptr[2]));
|
263
|
+
rb_ary_store(results, 3, DBL2NUM(params_ptr[3]));
|
264
|
+
RB_GC_GUARD(params);
|
265
|
+
RB_GC_GUARD(criterion);
|
264
266
|
return results;
|
265
267
|
}
|
266
268
|
|
@@ -375,10 +377,13 @@ find_split_params_reg(VALUE self, VALUE criterion, VALUE impurity, VALUE order,
|
|
375
377
|
split_opts_reg opts = { StringValuePtr(criterion), NUM2DBL(impurity) };
|
376
378
|
VALUE params = na_ndloop3(&ndf, &opts, 3, order, features, targets);
|
377
379
|
VALUE results = rb_ary_new2(4);
|
378
|
-
|
379
|
-
rb_ary_store(results,
|
380
|
-
rb_ary_store(results,
|
381
|
-
rb_ary_store(results,
|
380
|
+
double* params_ptr = (double*)na_get_pointer_for_read(params);
|
381
|
+
rb_ary_store(results, 0, DBL2NUM(params_ptr[0]));
|
382
|
+
rb_ary_store(results, 1, DBL2NUM(params_ptr[1]));
|
383
|
+
rb_ary_store(results, 2, DBL2NUM(params_ptr[2]));
|
384
|
+
rb_ary_store(results, 3, DBL2NUM(params_ptr[3]));
|
385
|
+
RB_GC_GUARD(params);
|
386
|
+
RB_GC_GUARD(criterion);
|
382
387
|
return results;
|
383
388
|
}
|
384
389
|
|
@@ -464,8 +469,10 @@ find_split_params_grad_reg
|
|
464
469
|
double opts[3] = { NUM2DBL(sum_gradient), NUM2DBL(sum_hessian), NUM2DBL(reg_lambda) };
|
465
470
|
VALUE params = na_ndloop3(&ndf, opts, 4, order, features, gradients, hessians);
|
466
471
|
VALUE results = rb_ary_new2(2);
|
467
|
-
|
468
|
-
rb_ary_store(results,
|
472
|
+
double* params_ptr = (double*)na_get_pointer_for_read(params);
|
473
|
+
rb_ary_store(results, 0, DBL2NUM(params_ptr[0]));
|
474
|
+
rb_ary_store(results, 1, DBL2NUM(params_ptr[1]));
|
475
|
+
RB_GC_GUARD(params);
|
469
476
|
return results;
|
470
477
|
}
|
471
478
|
|
@@ -497,6 +504,9 @@ node_impurity_cls(VALUE self, VALUE criterion, VALUE y_nary, VALUE n_elements_,
|
|
497
504
|
|
498
505
|
xfree(histogram);
|
499
506
|
|
507
|
+
RB_GC_GUARD(y_nary);
|
508
|
+
RB_GC_GUARD(criterion);
|
509
|
+
|
500
510
|
return ret;
|
501
511
|
}
|
502
512
|
|
@@ -531,6 +541,8 @@ node_impurity_reg(VALUE self, VALUE criterion, VALUE y)
|
|
531
541
|
|
532
542
|
xfree(sum_vec);
|
533
543
|
|
544
|
+
RB_GC_GUARD(criterion);
|
545
|
+
|
534
546
|
return ret;
|
535
547
|
}
|
536
548
|
|
data/lib/rumale.rb
CHANGED
@@ -30,10 +30,12 @@ require 'rumale/linear_model/linear_regression'
|
|
30
30
|
require 'rumale/linear_model/ridge'
|
31
31
|
require 'rumale/linear_model/lasso'
|
32
32
|
require 'rumale/linear_model/elastic_net'
|
33
|
+
require 'rumale/linear_model/nnls'
|
33
34
|
require 'rumale/kernel_machine/kernel_svc'
|
34
35
|
require 'rumale/kernel_machine/kernel_pca'
|
35
36
|
require 'rumale/kernel_machine/kernel_fda'
|
36
37
|
require 'rumale/kernel_machine/kernel_ridge'
|
38
|
+
require 'rumale/kernel_machine/kernel_ridge_classifier'
|
37
39
|
require 'rumale/multiclass/one_vs_rest_classifier'
|
38
40
|
require 'rumale/nearest_neighbors/vp_tree'
|
39
41
|
require 'rumale/nearest_neighbors/k_neighbors_classifier'
|
@@ -59,6 +61,10 @@ require 'rumale/ensemble/random_forest_classifier'
|
|
59
61
|
require 'rumale/ensemble/random_forest_regressor'
|
60
62
|
require 'rumale/ensemble/extra_trees_classifier'
|
61
63
|
require 'rumale/ensemble/extra_trees_regressor'
|
64
|
+
require 'rumale/ensemble/stacking_classifier'
|
65
|
+
require 'rumale/ensemble/stacking_regressor'
|
66
|
+
require 'rumale/ensemble/voting_classifier'
|
67
|
+
require 'rumale/ensemble/voting_regressor'
|
62
68
|
require 'rumale/clustering/k_means'
|
63
69
|
require 'rumale/clustering/mini_batch_k_means'
|
64
70
|
require 'rumale/clustering/k_medoids'
|
@@ -77,6 +83,7 @@ require 'rumale/manifold/tsne'
|
|
77
83
|
require 'rumale/manifold/mds'
|
78
84
|
require 'rumale/metric_learning/fisher_discriminant_analysis'
|
79
85
|
require 'rumale/metric_learning/neighbourhood_component_analysis'
|
86
|
+
require 'rumale/metric_learning/mlkr'
|
80
87
|
require 'rumale/neural_network/adam'
|
81
88
|
require 'rumale/neural_network/base_mlp'
|
82
89
|
require 'rumale/neural_network/mlp_regressor'
|
@@ -97,6 +104,7 @@ require 'rumale/preprocessing/one_hot_encoder'
|
|
97
104
|
require 'rumale/preprocessing/ordinal_encoder'
|
98
105
|
require 'rumale/preprocessing/binarizer'
|
99
106
|
require 'rumale/preprocessing/polynomial_features'
|
107
|
+
require 'rumale/preprocessing/kernel_calculator'
|
100
108
|
require 'rumale/model_selection/k_fold'
|
101
109
|
require 'rumale/model_selection/group_k_fold'
|
102
110
|
require 'rumale/model_selection/stratified_k_fold'
|
@@ -11,13 +11,15 @@ module Rumale
|
|
11
11
|
|
12
12
|
private
|
13
13
|
|
14
|
-
def enable_linalg?
|
14
|
+
def enable_linalg?(warning: true)
|
15
15
|
if defined?(Numo::Linalg).nil?
|
16
|
-
warn('If you want to use features that depend on Numo::Linalg, you should install and load Numo::Linalg in advance.')
|
16
|
+
warn('If you want to use features that depend on Numo::Linalg, you should install and load Numo::Linalg in advance.') if warning
|
17
17
|
return false
|
18
18
|
end
|
19
19
|
if Numo::Linalg::VERSION < '0.1.4'
|
20
|
-
|
20
|
+
if warning
|
21
|
+
warn('The loaded Numo::Linalg does not implement the methods required by Rumale. Please load Numo::Linalg version 0.1.4 or later.')
|
22
|
+
end
|
21
23
|
return false
|
22
24
|
end
|
23
25
|
true
|
data/lib/rumale/dataset.rb
CHANGED
@@ -12,22 +12,26 @@ module Rumale
|
|
12
12
|
# Load a dataset with the libsvm file format into Numo::NArray.
|
13
13
|
#
|
14
14
|
# @param filename [String] A path to a dataset file.
|
15
|
+
# @param n_features [Integer/Nil] The number of features of data to load.
|
16
|
+
# If nil is given, it will be detected automatically from given file.
|
15
17
|
# @param zero_based [Boolean] Whether the column index starts from 0 (true) or 1 (false).
|
16
18
|
# @param dtype [Numo::NArray] Data type of Numo::NArray for features to be loaded.
|
17
19
|
#
|
18
20
|
# @return [Array<Numo::NArray>]
|
19
21
|
# Returns array containing the (n_samples x n_features) matrix for feature vectors
|
20
22
|
# and (n_samples) vector for labels or target values.
|
21
|
-
def load_libsvm_file(filename, zero_based: false, dtype: Numo::DFloat)
|
23
|
+
def load_libsvm_file(filename, n_features: nil, zero_based: false, dtype: Numo::DFloat)
|
22
24
|
ftvecs = []
|
23
25
|
labels = []
|
24
|
-
|
26
|
+
n_features_detected = 0
|
25
27
|
CSV.foreach(filename, col_sep: "\s", headers: false) do |line|
|
26
28
|
label, ftvec, max_idx = parse_libsvm_line(line, zero_based)
|
27
29
|
labels.push(label)
|
28
30
|
ftvecs.push(ftvec)
|
29
|
-
|
31
|
+
n_features_detected = max_idx if n_features_detected < max_idx
|
30
32
|
end
|
33
|
+
n_features ||= n_features_detected
|
34
|
+
n_features = [n_features, n_features_detected].max
|
31
35
|
[convert_to_matrix(ftvecs, n_features, dtype), Numo::NArray.asarray(labels)]
|
32
36
|
end
|
33
37
|
|